Unlocking AI Potential: Leveraging Real-Time Data Streaming – Prabhu Patel
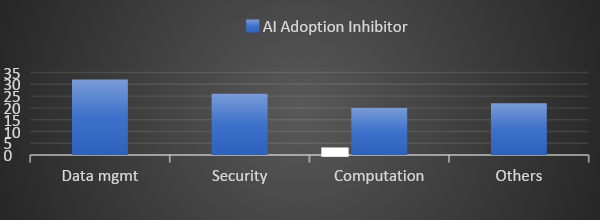
In 2023, the S&P Global Market Intelligence and Commission conducted a global survey of more than 1500 AI practitioners and published a report about the challenges of AI sustainability and data infrastructure. The study outlines the challenges and opportunities encountered during organizations’ AI journeys. The access to reliable data is the most significant barrier to AI innovation. A great AI/ML model is essential, but high-quality, trustworthy & clean data is uber important. The success of organizations in AI will depend heavily on their data infrastructure.[1]
According to the survey, data management emerges as the primary obstacle, constituting a substantial 32% of the challenges encountered in the domain of AI and ML. This revelation eclipses concerns pertaining to security, which garnered 26% of responses, and compute performance, trailing behind at 20%. Such insights serve as a clarion call for organizations to realign their focus towards fortifying their data architectures, laying the groundwork necessary to harness the full potential of the AI revolution.
The saying “you get out what you put in” holds a lot of truth when it comes to AI. This means that the quality of input data directly affects the accuracy and effectiveness of the AI output. In order for AI to be stable and sustainable, it’s crucial to have accurate, reliable, and regularly updated data. Just like a curious reader is always looking for new information to expand their knowledge, AI systems need to constantly take in new data and integrate it with existing knowledge to promote adaptive learning and growth.
Data-in-motion holds an advantage over traditional data-at-rest architecture.
These include:
1) Real-time Decision Making: Data streaming permits AI structures to manner statistics because it arrives, taking into consideration real-time selection-making. This is vital in applications like fraud detection, cybersecurity, and independent automobiles, in which selections want to be made immediately based at the maximum current information.
2) Continuous Learning: With records streaming, AI models can constantly replace and enhance themselves as new statistics turns into available. This facilitates adaptive mastering, where models can modify to changing styles and behaviors without the want for periodic retraining.
3) Scalability: Streaming architectures can easily scale to handle massive volumes of facts from numerous resources, that is critical for AI packages managing big datasets along with social media analytics, sensor statistics, or monetary transactions.
4) Reduced Latency: By processing records in real-time, records streaming reduces latency as compared to batch processing. This is critical in applications wherein even a mild delay can have large outcomes, including in algorithmic buying and selling or healthcare tracking structures.
5) Anomaly Detection: AI models skilled on streaming facts can continuously monitor for anomalies or deviations from everyday patterns. This is valuable for detecting fraud, community intrusions, or device failures in industrial settings.
6) Personalization: Streaming records can be used to customise consumer experiences in real-time. AI fashions can analyze user behavior as it happens and offer customized recommendations, content material, or commercials thus.
7) Dynamic Adaptation: With streaming statistics, AI structures can adapt dynamically to adjustments of their environment. For example, in clever grid systems, AI algorithms can modify electricity distribution based totally on real-time call for and supply fluctuations.
To fully utilize the potential of AI, organizations need to focus on improving data accessibility. One effective approach is to establish a real-time data mesh that facilitates data access throughout the organization. This allows AI teams to access and utilize data sets without requiring extensive coordination with data owners or setting up complex integrations. By separating data product ownership from AI consumption, organizations create an environment where AI can deliver actionable insights more efficiently. This streamlined process accelerates the return on investment (ROI) for AI initiatives.[2]
References:
[1 ]S&P Global Market Intelligence, 2023 Global Trends in AI Report, August, 2023
https://www.weka.io/trends-in-ai/
[2] https://www.confluent.io/blog/maximizing-the-power-of-ai-with-data-streaming/